Welcome to the Meta-Analysis of Economics Research (MAER) Network.
MAER-Net is an international network of scholars committed to improving economic science through meta-analysis. The purpose of our network is to serve as a clearinghouse for research in meta-analysis and economics and to improve the communication among scholars in this rapidly growing field.
The MAER-Net
Community.
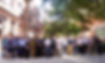
The MAER-Net Colloquium is the annual meeting of our group to exchange about new meta-analysis application and methodological advances.
Find reporting guidelines for meta-analysis research in economics and publications of the MAER-Net community.
The MAER-Net blog presents discussions and posts about recent research and advances in meta-analysis research.
ABOUT META-REGRESSION ANALYSIS
Why Meta?
In an era characterized by the rapid expansion of research publications and a flood of empirical findings on any given subject, knowledge and sensible policy action are being drowned. All reviews, whether conventional or systematic, are vulnerable to publication selection bias. Without some objective way to integrate this sea of research results, ideology and self-interest will dominate the public discussion of economic research. What we need is balanced and critical methodology to integrate diverse research findings and to reveal the nuggets of ‘truth’ that have settled to the bottom. This is precisely what Meta-Regression Analysis (MRA) can do and has done!
.jpg)
What is MRA?
MRA is the statistical analysis of previously reported regression results (Stanley and Jarrell, 1989). It seeks to summarize and explain the disparate empirical findings routinely reported in nearly all areas of economics. Over the past decades, more than a thousand meta-analyses have been conducted in economics, with hundreds of new ones appearing each year.
Stanley and Jarrell (1989), Stanley (2001), Stanley and Doucouliagos (2012), and Doucouliagos (2016). Irsova et al. (2024) and the Journal of Economic Surveys offer meta-analysis guidelines for practitioners. These guidelines update previous introductions and recommendations to reflect the rapid advancement of meta-analysis methods. They are broadly accessible to scholars with little prior experience or formal training in meta-analysis. See Zuzana Irsova’s blog.
What have meta-analysts learned?
-
Economics research is highly underpowered: the sample size is often too small to find the underlying effect. A survey of 64,076 economic estimates from 159 areas of research and 6,700 empirical studies finds that the median statistical power is 18%, or less (Ioannidis et al, 2017), and it is only 5% at the Top 5 economics journals (Askarov et al., 2023b). Impotence begets bias.
-
Publication selection bias plagues most areas of economics research. Its effects often make a difference! Typically, reported economic effects are inflated by 100% with one-third inflated by a factor of four or more (Ioannidis et al, 2017).
-
Regression model misspecification is, in fact, the principal cause for the observed variation among reported economic findings, confirming the concerns famously expressed by Leamer (1983), Summers (1991), Sala-i-Martin (1997), and others.
-
The empirical literature often contains strong evidence against widely held economic theory and contrary to conventional narrative reviews (Stanley, 2001; Stanley, 2004; Doucouliagos and Stanley, 2009). Without some objective and systematic method of literature reviewing, conventional narrative reviews can draw any conclusions their authors wish.
-
Top economics journals are not only highly ‘selective’: they report research results that are more biased and more likely to be misleading than lower-ranked journals or unpublished papers (Askarov et al., 2023b). Specifically, nearly half of the reported statistically significant evidence is falsely positive when reported at Top 5 economics journals and two-thirds of this ‘positive’ evidence was selected to be positive. Fortunately, it is within the power of these economics journals to reduce biases and false positives if they choose to do so (Askarov et al., 2023a).
Publication selection inflation
-
"Many other commentators have addressed the issue of publication bias ... All agree that it is a serious problem" (Begg and Berlin, 1988, p. 421)
-
"Are all economic hypotheses false?” de Long and Lang (1992) rhetorically asked. Researchers and reviewers treat ‘statistically significant’ results more favorably; hence, they are more likely to be published. Studies that find relatively small and ‘insignificant’ effects are much less likely to be published, because they may be thought to say little about the phenomenon in question (Chopra et al., 2023). Publication selection bias is so strong that we are likely to be better off discarding 90% of the research results than to take them at face value (Stanley, Jarrell and Doucouliagos, 2010; Stanley, Doucouliagos and Ioannidis, 2017).
-
“(P)ublication bias is leading to a new formulation of Gresham’s law — like bad money, bad research drives out good” (Bland, 1988, p. 450).
Funnel graphs should look like this one, below, for the union-productivity literature, though they seldom do.
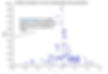.jpg)
-
Many economists have turned their attention to the issue of publication selection bias and have used meta-regression analysis to identify and correct it (Stanley, 2005; Rose and Stanley, 2005; Doucouliagos and Paldam, 2006; Disdier and Head, 2008; Doucouliagos and Stanley, 2009; Viscusi, 2015; Havránek, 2015; to cite a few early efforts). No other approach can cleanse the economic literature of the distorting effect of publication selection. We have developed methods that render this fundamental problem of empirical science mostly harmless when thoughtfully applied (Stanley, 2005; Stanley and Doucouliagos, 2014; Stanley et al., 2021; Bartoš et al., 2023a).
-
Recently, top economics journals have joined our movement of identifying bias and selection in the economics research record by publishing new methods to identify publication selection bias and its components (e.g. p-hacking) (Brodeur et al., 2016, 2020 & 2023; Andrews and Kasy, 2019; Elliott et al., 2022). What many economists have not yet fully understood is that p-hacking is just another flavor of the same problem of publication selection bias that meta-analysts have been addressing for decades.
Selected MAER-Net references
Andrews, I., & Kasy, & M. (2019). Identification of and correction for publication bias. American Economic Review, 109(8), 2766–2794.
Askarov, Z., Doucouliagos A, and Doucouliagos H., and Stanley, T.D. (2023a). The significance of data-sharing policy. Journal of the European Economic Association, 21:1191–1226.
Askarov, Z., Doucouliagos A, and Doucouliagos H., and Stanley, T.D. (2023b). Selective and (mis)leading economics journals: Meta-research evidence. Journal of Economics Surveys.
Bartoš, F., Maier, M., Wagenmakers, E. J., Doucouliagos, H., & Stanley, T. D. (2023a). Robust Bayesian meta-analysis: Model averaging across complementary publication bias adjustment methods. Research Synthesis Methods, 14(1), 99–116.
Bartoš, F., Maier, M.,Wagenmakers, E. J., Nippold, F., Doucouliagos, H., Ioannidis, J. P. A., Otte,W.M., Sladekova, M., Deresssa, T. K., Bruns, S. B., Fanelli, D., & Stanley, T. D. (2023b). Footprint of publication selection bias on meta-analyses in medicine, environmental sciences, psychology, and economics. arXiv:2208.12334.
Bom, P. R. D., & Rachinger, H. (2019). A kinked meta-regression model for publication bias correction. Research Synthesis Methods, 10(4), 497–514.
Bom, P. R. D., & Rachinger, H. (2020). A generalized-weights solution to sample overlap in meta-analysis. Research Synthesis Methods, 11(6), 812–832.
Brodeur, A., Lé, M., Sangnier, M., & Zylberberg, Y. (2016). Star wars: The empirics strike back. American Economic Journal: Applied Economics, 8(1), 1–32.
Brodeur, A., Cook, N. &Heyes, A. (2020). Methods matter: P-hacking and causal inference in economics. American Economic Review, 110(11), 3634–3660.
Brodeur, A., Carrell, S., Figlio, D., & Lusher, L. (2023). Unpacking p-hacking and publication bias. American Economic Review, 113(11), 2974-3002.
Christensen, G. and Miguel, E. (2018). Transparency, reproducibility, and the credibility of economics research. Journal of Economic Literature 56.3, pp. 920–980.
Doucouliagos, H., Paldam, M. (2006). Aid effectiveness on accumulation: A meta study. Kyklos 59, 227-54.
Doucouliagos, H. and Stanley, T.D. (2009). Publication selection bias in minimum-wage research? A meta-regression analysis. British Journal of Industrial Relations 47, 406-28.
Elliott, G., Kudrin, N., & Wutrich, K. (2022). Detecting p-hacking. Econometrica 90(2), 887-906.
Fabo, B., Jancokova, M., Kempf, E., & Pastor, L. (2021). Fifty shades of QE: Comparing findings of central bankers and academics. Journal of Monetary Economics, 120:1-20.
Gechert, S. (2015). What fiscal policy is most effective? A meta-regression analysis. Oxford Economic Papers, 7, 553-80.
Gurevitch, J., Koricheva, J., Nakagawa, S., & Stewart, G. (2018). Meta-analysis and the science of research synthesis. Nature, 555, 175–182.
Havranek, T. (2015). Measuring intertemporal substitution: The importance of method choices and selective reporting. Journal of the European Economic Association, 13(6), 1180–1204.
Havranek, T., Irsova, Z., Laslopova, L., & Zeynalova, O. (2024). Publication and attenuation biases in measuring skill substitution. The Review of Economics and Statistics, forthcoming.
Havranek, T., Irsova, Z., & Zeynalova, O. (2018). Tuition fees and university enrolment: A meta-regression analysis. Oxford Bulletin of Economics and Statistics, 80(6), 1145–1184.
Havranek, T., Stanley, T. D., Doucouliagos, H., Bom, P., Geyer-Klingeberg, J., Iwasaki, I., Reed, W. R., Rost, K., & van Aert, R. C. M. (2020). Reporting guidelines for meta-analysis in economics. Journal of Economic Surveys, 34(3), 469–475.
Ioannidis, J., Stanley, T., & Doucouliagos, H. (2017). The power of bias in economics research. Economic Journal, 127(605), 236–265.
Irsova, Z., Doucouliagos, H., Havranek, T., Stanley, T.D. (2024). Meta-analysis of social science research: A practitioner’s guide. Journal of Economics Surveys, forthcoming.
Nakagawa, S., Yang, Y., Macartney, E. L., Spake, R., & Lagisz, M. (2023). Quantitative evidence synthesis: A practical guide on meta-analysis, meta-regression, and publication bias tests for environmental sciences. Environmental Evidence, 12 (8).
Rose, A.K., Stanley, T.D. (2005). A Meta-Analysis of the effect of common currency on international trade. Journal of Economic Surveys 19, 347-65.
Rusnak, M., Havranek, T., & Horvath, R. (2013). How to solve the price puzzle? A meta-analysis. Journal of Money, Credit and Banking, 45(1), 37–70.
Stanley, T.D. (2005). Beyond publication bias. Journal of Economic Surveys 19, 309-45.
Stanley, T.D. (2008). Meta-regression methods for detecting and estimating empirical effect in the presence of publication selection. Oxford Bulletin of Economics and Statistics 70, 103-127.
Stanley, T.D., Doucouliagos, C., 2012. Meta-Regression Analysis in Economics and Business. Routledge.
Stanley, T.D., Doucouliagos, C., 2014, Meta-regression approximations to reduce publication selection bias. Research Synthesis Methods 5, 60-78.
Stanley T.D. and Doucouliagos, H, 2017. Neither fixed nor random: Weighted least squares meta-regression analysis. Research Synthesis Methods 8, 19-42.
Stanley, T.D., Doucouliagos, C., Ioannidis, J.P.A., 2017. Finding the power to reduce publication bias. Statistics in Medicine 36, 1580-1598.
Stanley, T.D., Doucouliagos H., Ioannidis, J.P.A., and Carter, E. (2021). Detecting publication selection bias through excess statistical significance. Research Synthesis Methods, 12: 776-795.
RECENT BLOG POSTS
See all posts here